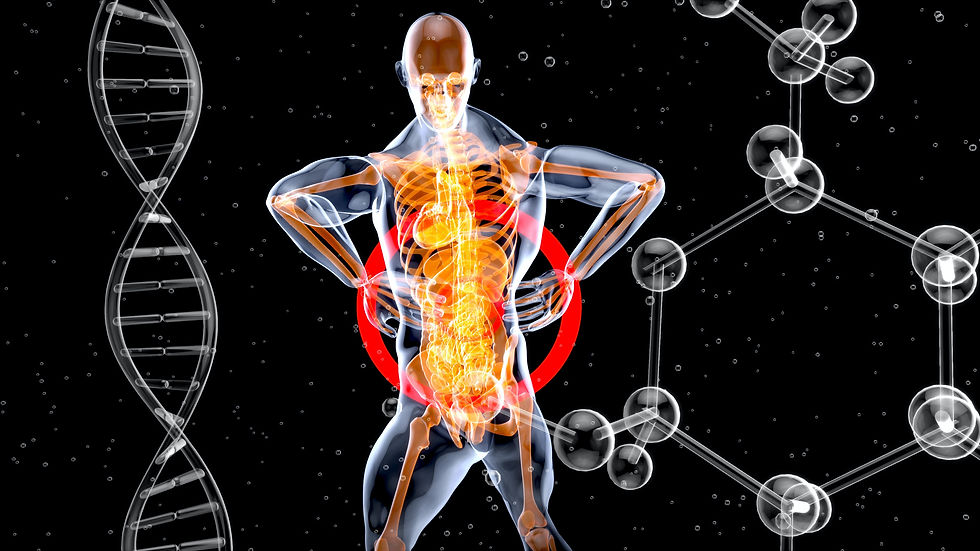
Healthcare is shifting from being reactive to proactive. An emerging trend is personalized healthcare using genomics, which is the study of structure, function, evolution, mapping, and editing of genomes; the clinical utility of genomics is the creation of a more complete health picture and a tailored care plan to the individual patient (Teng et al. 2014). Instead of a costly trial-and-error approach, applying genomics has the potential to save money by predicting drug responses and improving drug therapy selection (Teng et al., 2014). Minimizing the ambiguity about drug selection and dosage results in enhanced efficiency, which translates to lower costs (Teng et al., 2014). Moreover, the results from gene testing can facilitate conversations about genetic counselling and medical management to optimize patient outcomes (Teng et al., 2014). This paper will provide historical context of this trend, benefits and challenges associated with its use in the future, and questions for discussion.
Historical Elements of Genomics in Personalized Healthcare
Since the beginning of the 20th century, life expectancy has been steadily increasing (Cesuroglu et al., 2009). As a result of this aging population, the prevalence of chronic complex diseases has increased significantly, accounting for 84% of deaths and 76% of burden of disease in high- and upper-middle-income countries such as Canada (Cesuroglu et al., 2009). Complex diseases involve interactions with genes and lifestyle; common examples include cerebrovascular diseases, diabetes, osteoporosis, cancers, neurological conditions, and psychiatric disorders (Cesuroglu et al., 2009). Public health experts predict genomics in personalized healthcare will revolutionize how chronic complex diseases are managed in the 21st century (Cesuroglu et al. 2009). In the late 1990s and early 2000s, researchers began to imagine the implications of genomics as a widely used tool for prediction, diagnosis, and treatment (Bell, 1998). Precision medicine with genomics is useful for improving prevention, early intervention, diagnosis, and treatment of complex diseases (Cesuroglu et al., 2009). Genomics can identify variations in genetic structure known as polymorphisms, which indicate a predisposition for a disease (Cesuroglu et al., 2009). The successful completion of the Human Genome Project in 2003 was an important milestone as this information can be used to identify genes and polymorphisms that influence disease processes (Xu et al., 2008).
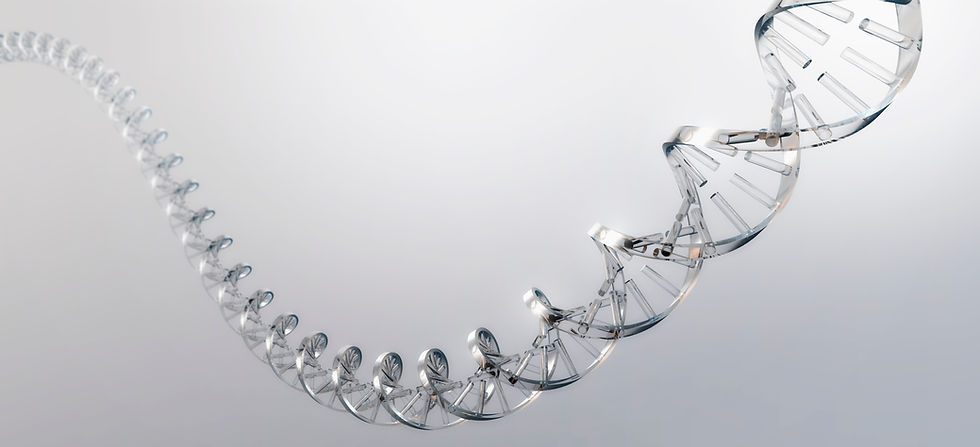
The Future of Genomics in Personalized Healthcare
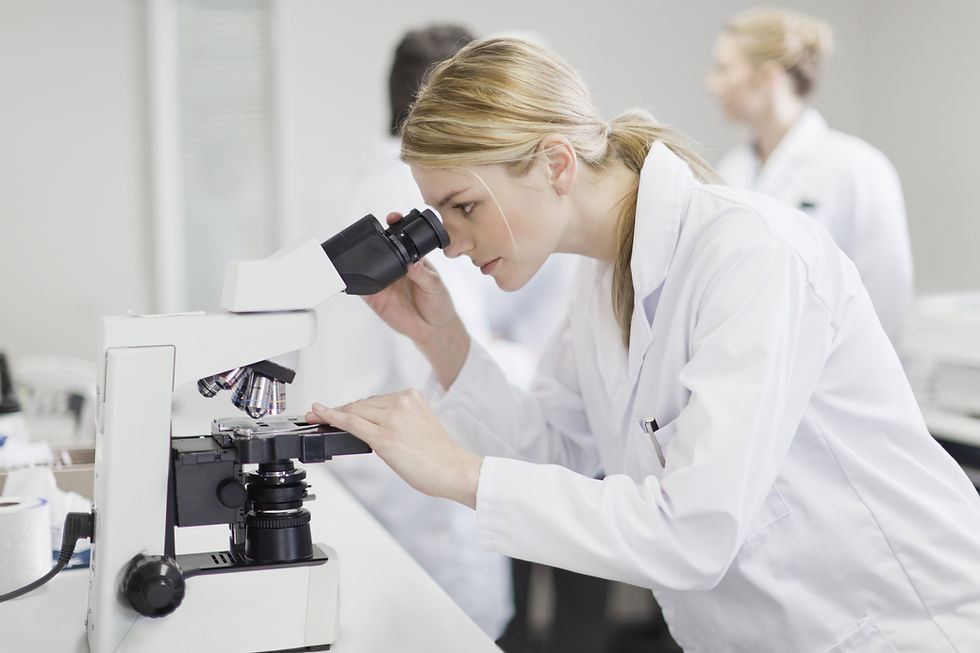
Chronic disease management accounts for approximately 75% of healthcare expenditures (Xu et al., 2008). Already strained healthcare systems will not be able to address the increasing societal and economic burden of chronic complex diseases without using an effective intervention model (Cesuroglu et al., 2009). Cesuroglu et al. (2009) likens genomics in personalized healthcare to vaccines for infectious diseases. A cost-effective intervention is needed to minimize disability and improve the quality of life in our aging population (Cesuroglu et al., 2009). The change in disease burden of our aging population influences the trend of genomics in personalized healthcare; additional conditions supporting this trend include technological advancements, which decrease costs, and data science innovations, which create meaningful outputs (Manamperi, 2008).
Personalized healthcare increases the likelihood of desirable health outcomes due to earlier disease intervention, better-targeted therapies, more informed clinical decisions, and decreased adverse medical reactions from medication or treatments (Teng et al., 2014). Using genomics to guide decisions will make wellness strategies more robust, and understanding pharmacogenetics ensures drug selection will be safer (Teng et al., 2014). Genomics will be a prediction tool and help monitor disease timing, progression, and prognosis (Teng et al., 2014).
Benefits of Genomics in Personalized Healthcare
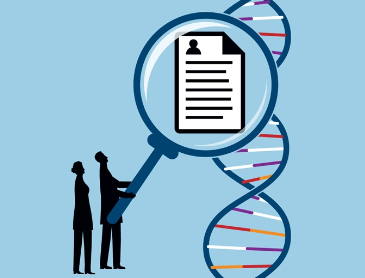
Ideally, a broad genotyping panel covering a wide range of known genetic variants involved in disease and therapy could be analyzed; however, the more genes that are tested the more costly the procedure (Xu et al., 2008). Costs can range from $300 to $2,000 USD for biomarker assays covering only a few genes (Xu et al., 2008). These costs will decline as advancements in technology and private companies make genetic analysis more accessible to people (Teng et al., 2014). For example, 23andMe (2022) boasts that 80% of their customers discover a meaningful genetic variant and 76% make healthier choices after receiving their report. The potential for businesses to make money on this trend has promoted investments and progress in the field of genomics (March, 2010). Moreover, drug companies are “armed with the blueprints of our genes” to design newer drugs with better targets (Manamperi, 2008). These improvements could lead to better medication compliance.
Researchers suggest that this trend will decrease hospital admission rates since there will be less adverse reactions or side effects when the disease management plan is individualized (Chawla & Davis, 2013). Moreover, if a patient is empowered and actively managing their disease then readmission rates should also decrease (Chawla & Davis, 2013). In fact, one study found that patients who received personalized healthcare had 36% fewer hospitalizations, 12.4% fewer emergency department visits, 6.5% reduction in total medical and pharmacy costs, and 18% fewer readmissions compared to a control group (Teng & Longworth, 2013).
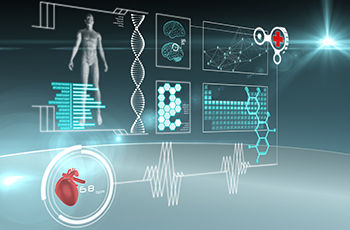
Challenges with Genomics in Personalized Healthcare
Genes alone do not predict complex diseases; non-genetic factors such as lifestyle and environment play a role in determining if and when a disease will develop (Collins et al., 2003). These gene-lifestyle interactions need to be considered otherwise there is a potential risk for this trend to exacerbate over diagnosis. Bulliard & Chiolero (2015) define over diagnosis as “the diagnosis of an abnormality that bears no substantial health hazard and no benefit for patients to be aware of.” The monitoring and interventions of over diagnosed conditions have financial implications on the healthcare system such as ongoing unnecessary diagnostic testing or screening (Bulliard & Chiolero, 2015).
Genomics has made it easier to identify genetic mutations, but understanding their clinical relevance is less understood (Loscalzo et al., 2007). According to Teng & Longworth (2013), “Science at the bench has far outpaced our understanding of how to broadly apply it at the bedside.” Although personalized healthcare is viewed as the optimal patient-centered approach, as the amount of patient data increases so does the complexity of interpreting all the information (Chawla & Davis, 2013). This poses as an operational challenge that can only be overcome with the help of artificial intelligence that is capable of aggregating and integrating significant amounts of data (Chawla & Davis, 2013). For example, the Collaborative Assessment and Recommendation Engine (CARE) system uses a filtering method and algorithm to build a detailed similarity model for a specific individual (Feldman & Chawla, 2014).
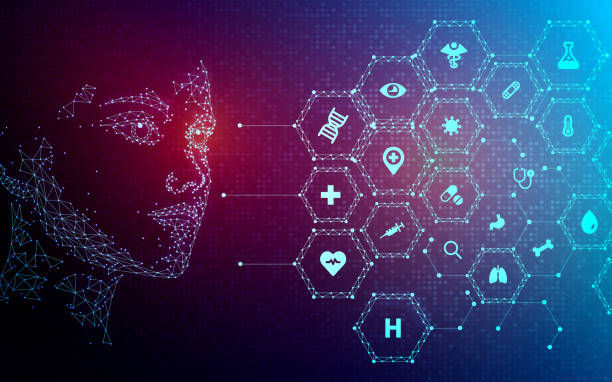
Questions Arising from Trends Analysis
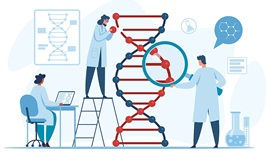
Bibliometrics related to genomics in academic literature and case studies in healthcare settings demonstrate an increasing popularity of this trend over the past decade. Analyzing this trend required research into the contextual factors that influence healthcare leaders and policy makers, which revealed a risk for health inequities. According to Kaye et al. (2001), policy makers in developing countries must improve infrastructure, educate the public about genomics, and enable partnerships between research and industrial sectors with institutes or organizations. There will be pressure over time to deliver DNA sequencing technologies at lower costs so it is not prohibitively expensive (Teng & Longworth, 2013). An unanswered question of applying genomics in public health is what are the ethical and social issues that can arise? Similarly, there are likely to be legal consequences of genetic screening protocols. This requires further consideration into the safeguards that will protect the public from manipulation of genomic data or breaches of confidentiality.
Conclusion
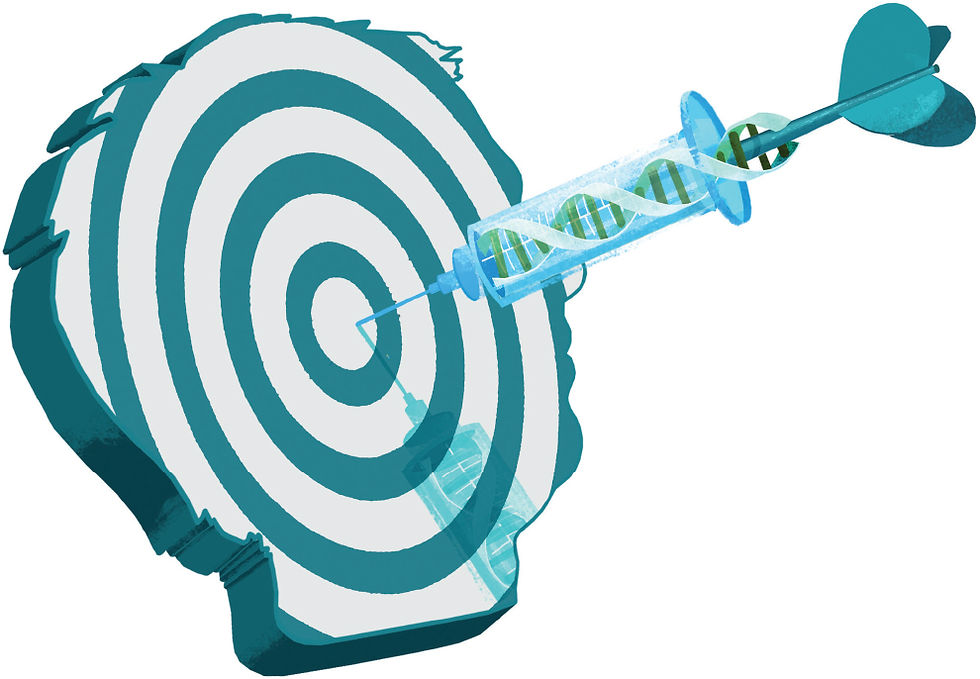
The vision of personalized healthcare requires healthcare teams to focus on specific characteristics at the individual level; exploring personal health data, genetic information, and lifestyle factors ensures risk assessments are thorough and intervention recommendations will be relevant and effective (Cesuroglu et al., 2009). March (2010) states “Personalized healthcare aims to deliver the right treatment, to the right patient, at the right dose and at the right (the first) time.” The trend of genomics in personalized healthcare will transform how we provide care and define health; instead of health being the absence of disease, this new approach will emphasize preventing and managing chronic illnesses to maintain good health (Xu et al., 2008). The potential for incorporating genomics into routine healthcare practices will optimize healthcare by minimizing the risks of life-threating and debilitating diseases, whilst promoting healthy choices for each individual. Ask yourself, what changes would you make if you knew the diseases you were at risk for developing before you became symptomatic? Do you agree that you’d prefer a personalized plan to manage your health? This revolutionary approach valuing a care delivery model that is patient-centric is possible with the use of genomics in personalized healthcare.
Bell, J. (1998). The new genetics in clinical practice. British Medical Journal, 316(7131), 618- 620. https://doi.org/10.1136/bmj.316.7131.618
Bulliard, J.L. & Chiolero, A. (2015). Screening and overdiagnosis: Public health implications. Public Health Reviews, 36(8), 1-5. https://doi.org/10.1186/s40985-015-0012-1
Cesuroglu, T., Karaca, S. & Erge, S. (2009). A practical model for personalized healthcare with a public health genomics perspective. Personalized Medicine, 5(5), 1-9. https://doi.org/10.2217/pme.09.37 Chawla, N.V. & Davis, D.A. (2013). Bringing big data to personalized healthcare: A patient-centered framework. Journal of General Internal Medicine, 28(3), 660-665. https://doi.org/10.1007/s11606-013-2455-8
Collins, F.S., Green, E.D., Guttmacher, A.E. & Guyer, M.S. (2003). A vision for the future of genomic research. Nature, 422, 835-847. https://doi.org/10.1038.nature01626 Feldman, K. & Chawla, N.V. (2014). Scaling personalized healthcare with big data. 2nd International Conference on Big Data and Analytics in Healthcare, Singapore.
Kaye, C.I., Laxova, R. & Livingston, J.E. (2001). Integrating genetic services into public health: Guidance for state and territorial programs from the National Newborn Screening and Genetics Resources Center. Community Genetics, 4, 175-196.
https://doi.org/10.1159/000051179 Loscalzo, J., Kohane, I. & Barabasi, A.L. (2007). Human disease classification in the postgenomic era: A complex systems approach to human pathobiology. Molecular Systems Biology, 3(1), 124. https://doi.org/10.1038/msb4100163 Manamperi, A. (2008). Current developments in genomics and personalized health care: Impact on public health. Asia-Pacific Journal of Public Health, 20(3), 242-250. https://doi.org/10.1177/1010539508316783 March, R. (2010). Delivering on the promise of personalized healthcare. Personalized Medicine, 7(3), 1-11. https://doi.org/10.2217/pme.10.17
Teng, T., Eng, C., Hess, C.A., Holt, M.A., Moran, R.T., Sharp, R.R., Traboulsi, E.I. (2014). Building an innovative model for personalized healthcare. Cleveland Clinic Journal of Medicine, 79(1), 1- 9. https://doi.org/10.3949/ccjm.79s1.01 Teng, K.A. & Longworth, D.L. (2013). Personalized healthcare in the era of value-based
healthcare. Personalized Medicine, 10(3), 1-8. https://doi.org/10.2217/pme.13.14 Xu, L.H., Zheng, H. Sedmak, D.D. & Sadee, W. (2008). The re-emerging concept of personalized healthcare. Personalized Medicine, 5(5), 1-11. https://doi.org/10.2217/17410541.5.5.257 23andMe Inc (2022). Know your genes. Own your health. 23andMe. Retrieved from https://www.23andme.com/en-ca/
Comments